03/21
2022
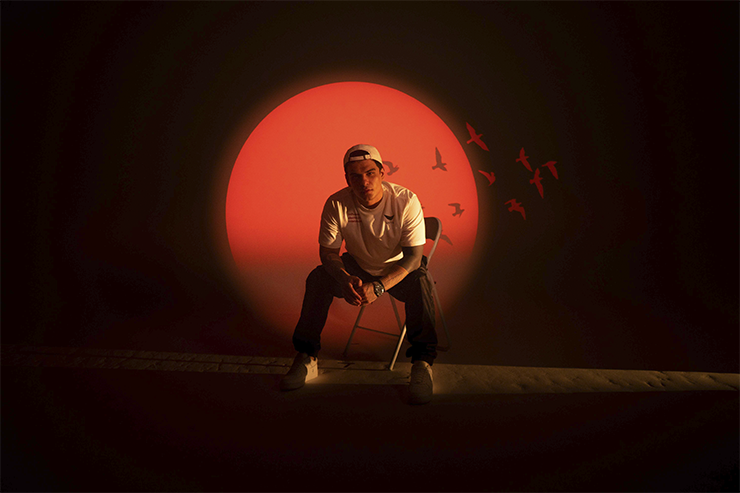
Vintage Culture drops new single ‘Commotion’ with Maxi Jazz
Maxi Jazz, the legendary vocalist and co-founder of seminal recording group Faithless, has joined forces with Vintage Culture, on a new dance classic ‘Commotion’. Vintage Culture has also unveiled a sprawling world tour, titled EARTH Tour and Coachella set.